Are you gearing up for a career shift or aiming to ace your next interview? Look no further! We’ve curated a comprehensive guide to help you crack the interview for the coveted AI Engineer position. From understanding the key responsibilities to mastering the most commonly asked questions, this blog has you covered. So, buckle up and let’s embark on this journey together
Acing the interview is crucial, but landing one requires a compelling resume that gets you noticed. Crafting a professional document that highlights your skills and experience is the first step toward interview success. ResumeGemini can help you build a standout resume that gets you called in for that dream job.
Essential Interview Questions For AI Engineer
1. Can you explain the concept of overfitting and underfitting in machine learning models, and how do you identify and address these issues?
Overfitting occurs when a model is too complex and learns the training data too well, leading to poor performance on unseen data. Underfitting occurs when a model is too simple and fails to capture the underlying patterns in the data.
- Overfitting can be identified by observing high training accuracy but low test accuracy.
- Underfitting can be identified by observing low training and test accuracy.
To address overfitting, techniques such as regularization, early stopping, and dimensionality reduction can be used. To address underfitting, increasing model complexity, gathering more data, or feature engineering can be employed.
2. How do you approach the task of feature engineering for a machine learning project?
Exploratory Data Analysis
- Understand the business problem and data.
- Explore the data to identify patterns, relationships, and outliers.
Feature Transformation
- Create new features from existing ones using mathematical operations, binning, or encoding.
- Remove redundant or irrelevant features.
Feature Selection
- Identify the most informative features using techniques like correlation analysis or feature importance scores.
- Select a subset of features that maximize model performance while minimizing overfitting.
3. Explain the process of designing and implementing a deep learning model for image classification.
- Define the problem and collect a suitable dataset.
- Choose an appropriate deep learning model architecture, such as CNN or ResNet.
- Preprocess the images by resizing, normalizing, or applying data augmentation techniques.
- Train the model using an optimizer and a loss function.
- Evaluate the model’s performance using metrics like accuracy, precision, and recall.
- Tune hyperparameters and improve model performance through techniques like dropout or regularization.
4. Discuss the challenges associated with training and deploying a large-scale NLP model.
- Data Availability and Quality: Acquiring and cleaning large amounts of text data.
- Computational Resources: Training large NLP models requires significant GPU power and memory.
- Model Complexity: Large models are prone to overfitting and require careful regularization techniques.
- Interpretability: Understanding and explaining the predictions made by large NLP models can be challenging.
- Deployment and Scalability: Deploying and scaling large NLP models for real-time applications can be complex.
5. How do you ensure the fairness and ethical considerations when developing and deploying AI models?
- Bias Mitigation: Identify and address biases in training data and model algorithms.
- Transparency and Explainability: Ensure models are transparent and their decisions can be explained.
- Privacy and Security: Protect user data and privacy while developing and deploying AI models.
- Accountability: Establish mechanisms for holding AI systems and their developers accountable for their impact.
- Collaboration with Ethicists: Involve ethicists in the design and development process to ensure ethical considerations are met.
6. What are the key factors to consider when evaluating the performance of a machine learning model?
- Accuracy: The overall correctness of the model’s predictions.
- Precision and Recall: The ability of the model to correctly identify specific classes.
- F1-Score: A balanced measure of precision and recall.
- AUC-ROC: The area under the receiver operating characteristic curve.
- Confusion Matrix: Provides insights into the types of errors made by the model.
- Cross-Validation: Ensures the model’s performance is not biased towards a particular dataset.
7. How do you handle missing values in a dataset intended for machine learning?
- Imputation: Filling in missing values with estimated or derived values.
- Deletion: Removing rows or columns with missing values if they do not significantly impact the model.
- Multiple Imputation: Generating multiple plausible values for missing data and using them in multiple models.
- Indicator Variables: Creating binary features to indicate the presence or absence of missing values.
- Model Selection: Choosing models that can handle missing values, such as decision trees or regularized regression.
8. Explain the difference between supervised and unsupervised learning.
Supervised Learning
- Involves training a model on labeled data.
- Objective is to learn a mapping from input features to output labels.
- Examples: Classification, regression.
Unsupervised Learning
- Involves training a model on unlabeled data.
- Objective is to discover hidden patterns or structures in the data.
- Examples: Clustering, dimensionality reduction.
9. What are the advantages and disadvantages of using ensemble methods in machine learning?
Advantages
- Improved accuracy and robustness.
- Reduced variance and overfitting.
- Ability to combine different models and learning algorithms.
Disadvantages
- Increased computational cost.
- Potential for overfitting if not carefully tuned.
- Difficulty in interpreting the results of ensemble models.
10. Describe your experience in working with cloud-based platforms for AI development.
- Experience with cloud platforms such as AWS, Azure, or GCP.
- Familiarity with cloud services for AI, such as ML Engine, Azure Machine Learning, or SageMaker.
- Understanding of cloud-based infrastructure for training and deploying AI models.
- Ability to leverage cloud resources for scalability, cost optimization, and collaboration.
Interviewers often ask about specific skills and experiences. With ResumeGemini‘s customizable templates, you can tailor your resume to showcase the skills most relevant to the position, making a powerful first impression. Also check out Resume Template specially tailored for AI Engineer.
Career Expert Tips:
- Ace those interviews! Prepare effectively by reviewing the Top 50 Most Common Interview Questions on ResumeGemini.
- Navigate your job search with confidence! Explore a wide range of Career Tips on ResumeGemini. Learn about common challenges and recommendations to overcome them.
- Craft the perfect resume! Master the Art of Resume Writing with ResumeGemini’s guide. Showcase your unique qualifications and achievements effectively.
- Great Savings With New Year Deals and Discounts! In 2025, boost your job search and build your dream resume with ResumeGemini’s ATS optimized templates.
Researching the company and tailoring your answers is essential. Once you have a clear understanding of the AI Engineer‘s requirements, you can use ResumeGemini to adjust your resume to perfectly match the job description.
Key Job Responsibilities
AI Engineers are responsible for designing, developing, and deploying artificial intelligence (AI) systems. They work with data scientists and other engineers to develop and implement AI solutions for a variety of industries. Some of the key responsibilities of an AI Engineer include:
1. Design and develop AI systems
AI Engineers design and develop AI systems that can perform a variety of tasks, such as image recognition, natural language processing, and machine learning. They work with data scientists to identify the data that is needed to train the AI system and develop the algorithms that will be used to process the data.
2. Implement AI systems
AI Engineers implement AI systems in a variety of environments, including cloud platforms, on-premises servers, and embedded devices. They work with system administrators to ensure that the AI system is properly installed and configured.
3. Monitor and maintain AI systems
AI Engineers monitor and maintain AI systems to ensure that they are running properly. They track the performance of the AI system and identify any potential issues. They also perform regular maintenance tasks to keep the AI system up-to-date.
4. Collaborate with other engineers and data scientists
AI Engineers collaborate with other engineers and data scientists to develop and implement AI solutions. They work with data scientists to identify the data that is needed to train the AI system and develop the algorithms that will be used to process the data. They also work with engineers to implement the AI system in a variety of environments.
Interview Tips
Preparing for an AI Engineer interview can be a daunting task, but there are a few things you can do to increase your chances of success. Here are a few tips to help you ace your interview:
1. Research the company and the position
Before you go to your interview, it is important to do your research on the company and the position. This will help you understand the company’s culture and values, as well as the specific requirements of the position. You can find information about the company on its website, social media pages, and Glassdoor. You can also find information about the position in the job description.
2. Practice your answers to common interview questions
There are a number of common interview questions that you are likely to be asked in an AI Engineer interview. These questions include:
- Tell me about your experience with AI.
- What are your strengths and weaknesses as an AI Engineer?
- Why are you interested in this position?
- What are your salary expectations?
- Do you have any questions for me?
It is important to practice your answers to these questions in advance so that you can deliver them confidently and concisely.
3. Be prepared to talk about your projects
In addition to practicing your answers to common interview questions, you should also be prepared to talk about your projects. This is a great way to demonstrate your skills and experience in AI. When talking about your projects, be sure to highlight the following:
- The problem that you were trying to solve.
- The approach that you took to solve the problem.
- The results that you achieved.
4. Be yourself
The most important thing is to be yourself in your interview. Companies are looking for people who are passionate about AI and who have the skills and experience to contribute to their team. If you are genuine and enthusiastic, you will be more likely to make a positive impression on the interviewers.
Next Step:
Now that you’re armed with interview-winning answers and a deeper understanding of the AI Engineer role, it’s time to take action! Does your resume accurately reflect your skills and experience for this position? If not, head over to ResumeGemini. Here, you’ll find all the tools and tips to craft a resume that gets noticed. Don’t let a weak resume hold you back from landing your dream job. Polish your resume, hit the “Build Your Resume” button, and watch your career take off! Remember, preparation is key, and ResumeGemini is your partner in interview success.
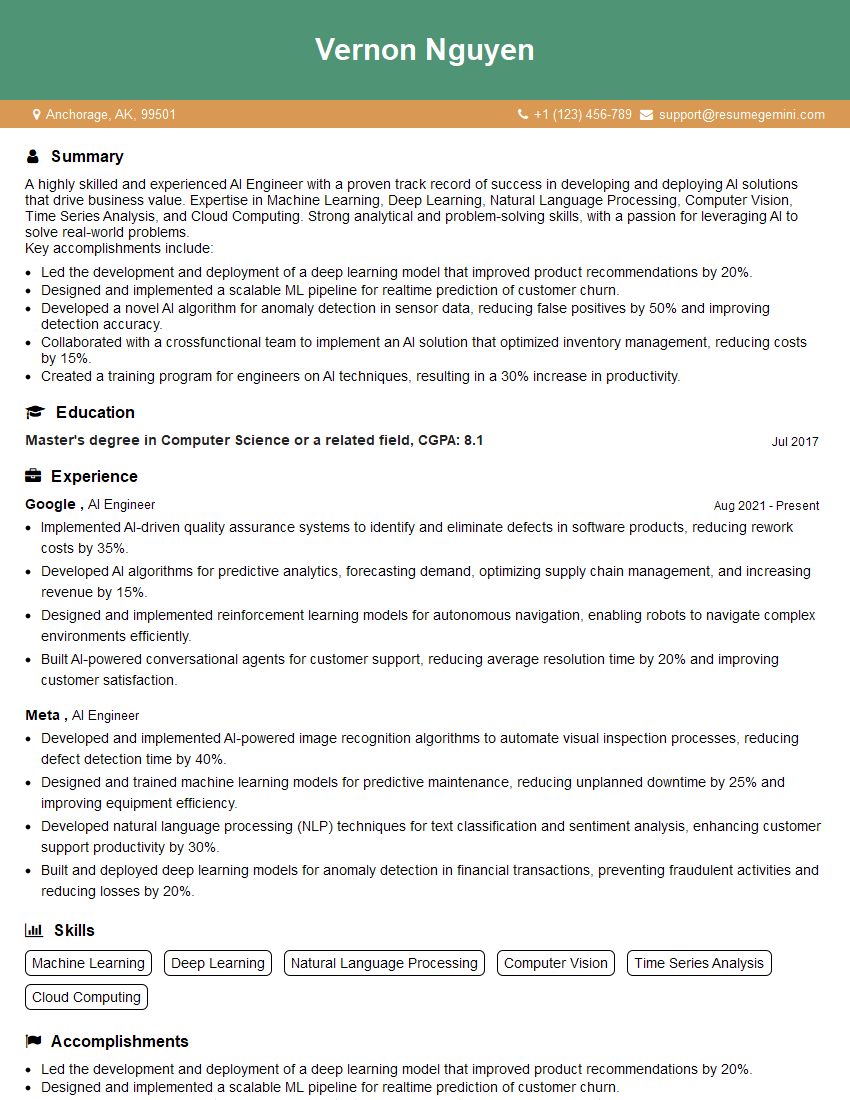